The MARBLE method is a fully unsupervised representation learning approach to obtain interpretable latent representations of neural dynamics. More generally, it introduces a statistical learning paradigm for non-linear dynamical systems based on a decomposition of the dynamical attractor into local flow fields. MARBLE representations achieve state-of-the-art decoding accuracy in neural dynamics and allow comparing computations across biological and artificial neural networks.
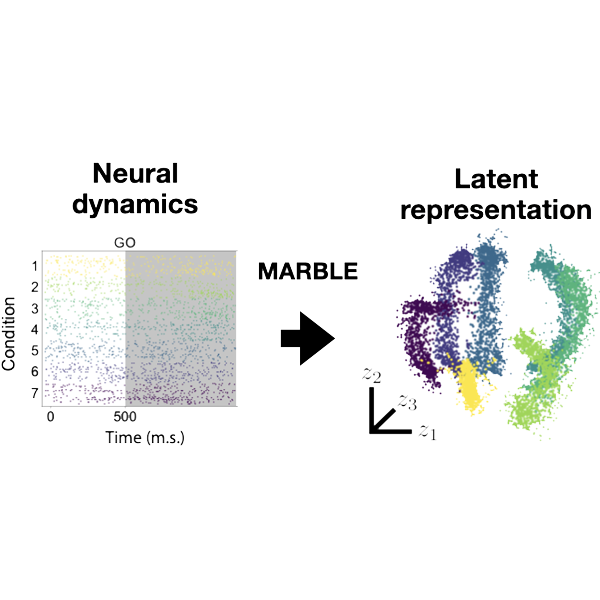